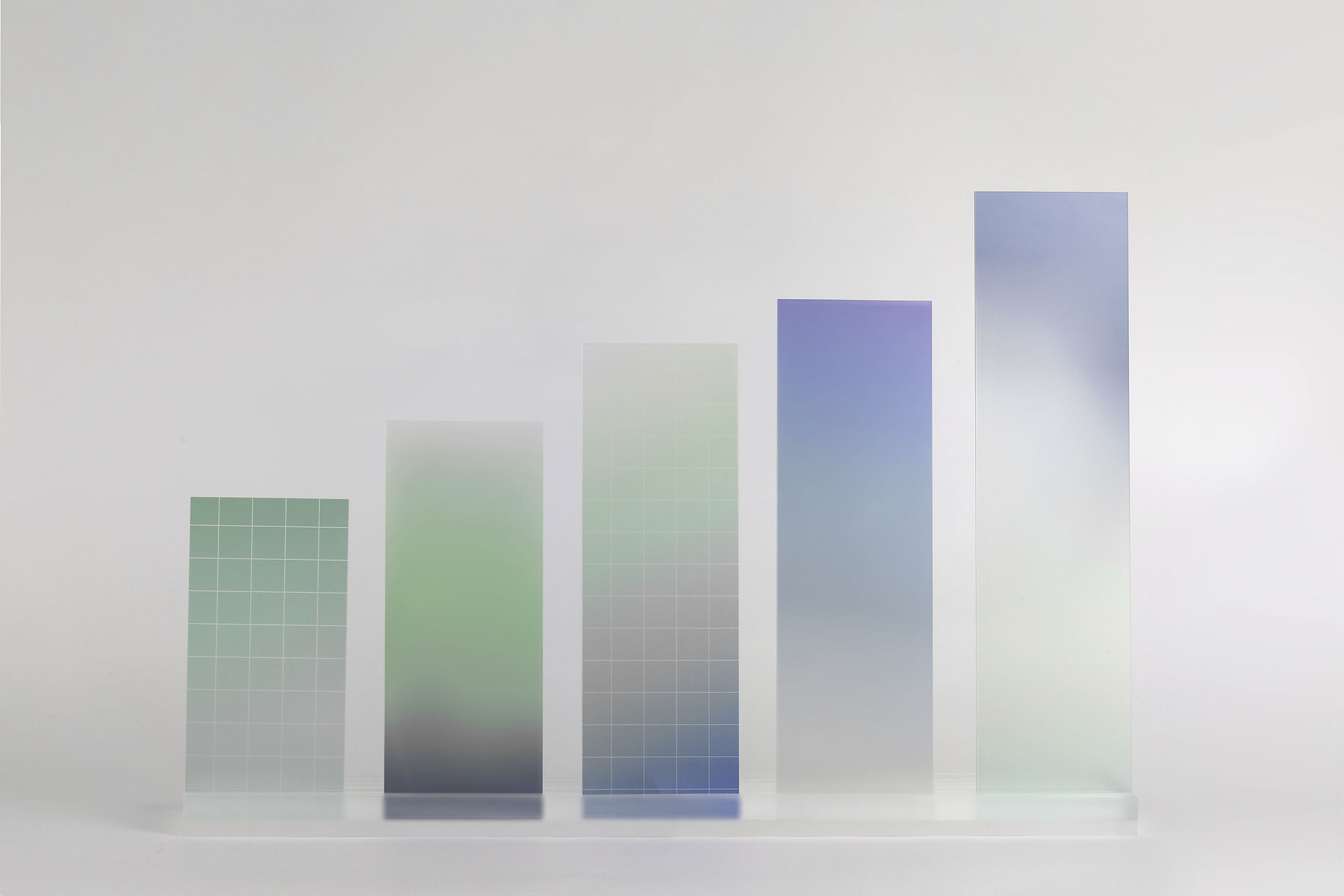
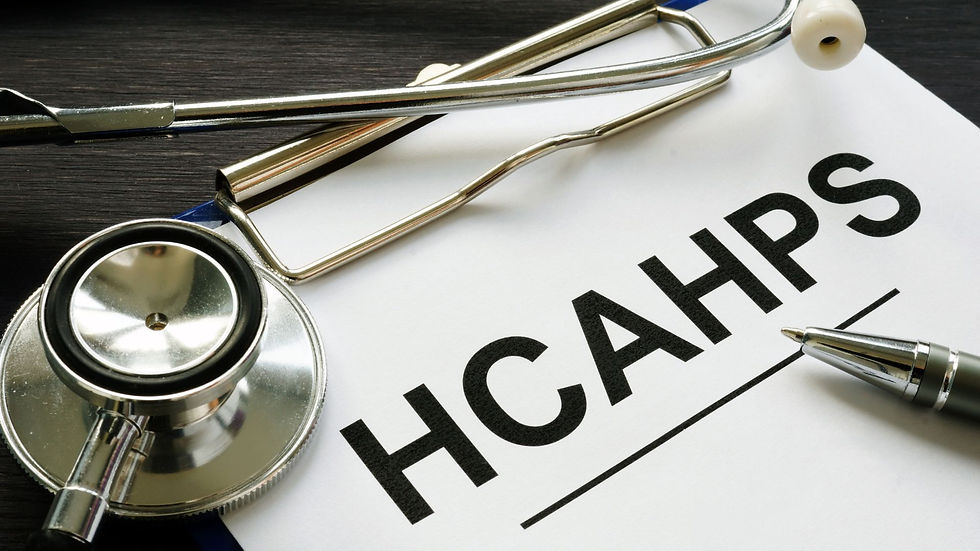
HCAHPS Patient Satisfaction Analysis (2013–2023)
Uncovering a Decade of Patient Feedback & Hospital Performance Trends
This project analyzes Hospital Consumer Assessment of Healthcare Providers and Systems (HCAHPS) data across U.S. hospitals from 2013 to 2023. By merging data from Maven Analytics (2013–2022) with the latest 2023 records from CMS HCAHPS, we create a comprehensive dataset capturing patient satisfaction trends, COVID-19 disruptions, and the strongest predictors of hospital ratings.
Through statistical modeling and interactive visualizations, this project provides data-driven insights for hospital leaders, researchers, and policymakers to improve healthcare quality and patient experiences.
Key Takeaways
What Matters Most?
Care Transition, Quietness, and Cleanliness have the strongest positive influence on hospital ratings.
Communication about Medicines consistently lags, particularly during pandemic years.
How Did COVID-19 Impact Patient Satisfaction?
Hospital ratings declined by approximately 2–3% between 2021–2022 due to staffing shortages, resource constraints, and care delays.
A partial recovery began in 2023, though some areas remain below pre-pandemic levels.
State-Level Differences Matter
Some states (e.g., North Dakota, Montana) improved despite the pandemic.
Others (e.g., Delaware, Hawaii) saw steep declines, highlighting regional disparities in hospital performance.
Key Features
Comprehensive Trends (2013–2023)
Tracks national and state-level hospital performance over a decade, offering a clear picture of patient sentiment over time.
Pandemic Impact Analysis
Reveals a 2–3% drop in patient satisfaction from 2021 to 2022, reflecting staffing shortages and care delays.
Shows a mild recovery in 2023, but some states and hospitals continue to struggle.
Identifying Key Drivers of Hospital Ratings
Lasso, Ridge, and Elastic Net regression models achieved R² > 0.93, highlighting the top measures that drive satisfaction.
SHAP analysis confirms that Care Transition, Cleanliness, and Quietness are the most influential predictors.
State-by-State Comparisons
Examines how different states performed before, during, and after COVID-19.
Identifies regional healthcare policies and hospital strategies that shaped patient experiences.
Visualizations & Reports
Explore trends via interactive dashboards and charts, including:
Yearly and measure-level trends (e.g., Communication, Cleanliness, Responsiveness).
Distribution of Bottom-box, Middle-box, and Top-box responses.
Feature importance plots showing what drives patient satisfaction the most.
Technologies Used
Python (Pandas, NumPy, Scikit-learn) – Data manipulation, cleaning, and modeling
Seaborn & Matplotlib – Creating visualizations
SHAP – Determining feature importance in machine learning models
Jupyter Notebooks – Organizing code and analysis in a structured workflow
Use Cases & Applications
For Hospital Leadership
Identify key areas for improvement (e.g., discharge protocols, patient communication).
Benchmark against state/national averages to track progress.
For Healthcare Researchers
Access historical and state-level performance data for evidence-based improvements.
Use predictive modeling and SHAP analysis to pinpoint actionable insights.
For Policy Makers
Examine regional disparities in healthcare quality.
Target resources and funding where patient satisfaction is lowest.
Project Workflow
Data Collection & Merging
2013–2022 Data: Maven Analytics Data Playground
2023 Data: CMS HCAHPS Archives
Processed using data_processor.ipynb, producing the final dataset for HCAHPS Patient Survey Analysis.ipynb.
Exploratory Data Analysis (EDA)
Examined Bottom-box, Middle-box, and Top-box percentages over time.
Investigated trends in key measures (e.g., Communication with Nurses, Responsiveness).
Analyzed COVID-19’s impact on hospital satisfaction scores.
Predictive Modeling & Insights
Built Lasso, Ridge, and Elastic Net regression models to predict Overall Hospital Rating.
Achieved R² > 0.93, ensuring high model accuracy.
Used SHAP to analyze feature importance, confirming that Care Transition, Cleanliness, and Quietness have the strongest impact.
Findings & Reporting
Communication About Medicines and Responsiveness were heavily impacted during COVID-19.
Findings & Insights
Pandemic Disruption
Hospital satisfaction dropped 2–3% in 2021–2022 due to staffing shortages, patient surges, and care delays.
Partial recovery in 2023, but some hospitals remain below pre-pandemic levels.
Core Drivers of Patient Satisfaction
Measure | Impact on Ratings |
Care Transition | Strongest positive effect |
Quietness | Highly significant |
Cleanliness | Strong correlation |
Communication About Medicines | Weakest-performing measure |
Responsiveness of Staff | Needs improvement |
State-Level Insights
Some states improved despite COVID-19, indicating strong policies and proactive leadership.
Others declined sharply due to resource shortages and healthcare disruptions.
Recommendations
1. Strengthen Care Transitions
Improve discharge processes to reduce confusion and enhance follow-ups.
Implement telehealth check-ins to ensure post-hospitalization care.
2. Enhance Patient Communication
Invest in medication counseling programs to reduce confusion about prescriptions.
Train staff to improve communication between patients, nurses, and doctors.
3. Maintain Clean & Quiet Environments
Ensure hospitals maintain high standards of cleanliness.
Minimize noise levels to create a more comfortable and healing environment.
4. Learn from High-Performing States
Analyze hospitals that improved during COVID-19.
Identify and implement their best practices to enhance patient satisfaction.
Conclusion
By uncovering the key drivers of hospital satisfaction, this project offers actionable insights for hospitals, researchers, and policymakers. Focusing on Care Transition, Quietness, and Cleanliness can significantly improve patient experiences and overall hospital ratings.
Explore interactive dashboards and notebooks for a deeper dive into the data!
GitHub: View Code & Notebooks
Questions or Collaboration?
Feel free to reach out! I’d be happy to discuss insights, improvements, or future research ideas.
Thank you for reading!
Project Gallery
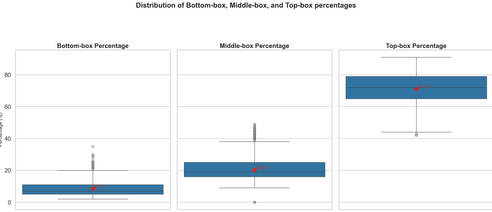
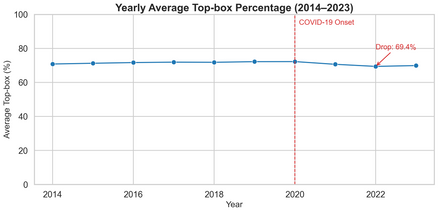


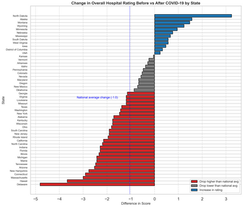
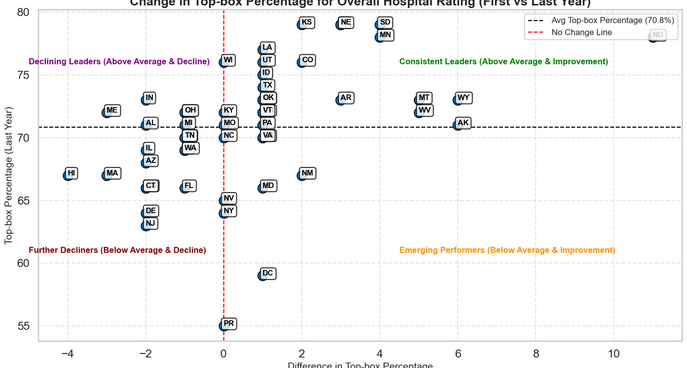
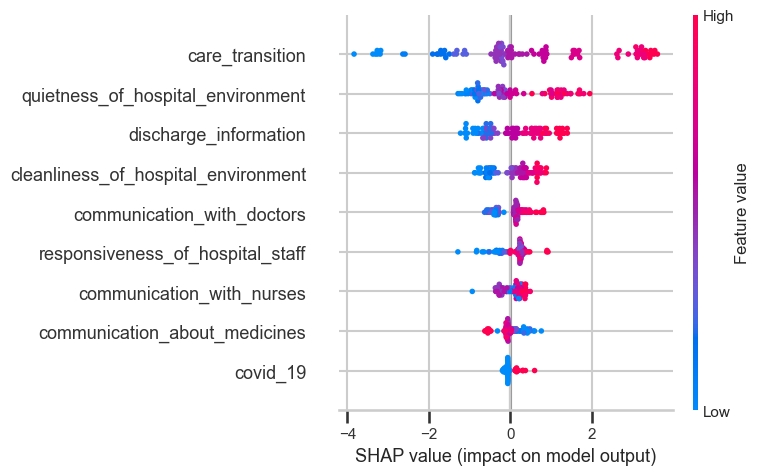